Due to a very busy week on the forum with a unusually high number of very difficult questions, plus work on the Enterprise DNA TV YouTube videos, I just didn’t have the time to put together a full entry on this challenge. However when I started putting my entry together, I pulled on what I think is a pretty interesting thread that I haven’t seen addressed in the other entries yet so I wanted to share it here.
My first thought in looking at the interview data was “are these data are reliable and valid”? ( Reliability addresses the consistency of a measure, and validity adddresses the accuracy of a measure. This graphic is a good illustration of the difference between reliability and validity:
In analyzing reliability and validity, I first created a numeric metric called Internal Consistency Score Squared (ICS Sq). For each interview, Unhappy was assigned a score of -1, Neutral = 0 and Happy = 1. I then took the difference of the Interview #1 and Interview #2 scores and then squared the difference, to put an increasing weight on larger deviations. ICS Sq has a max value of 4 (if one interview was scored Happy and the other Unhappy, or vice versa) and a min score of 0 (if the score for Interview #1 and #2 was the same). Every single step deviation (e.g, Unhappy to Neutral, Neutral to Happy, etc) gets a score of 1,
To assess reliability of the interview process, I compared the average ICS Sq for Interview #1 and Interview #2:
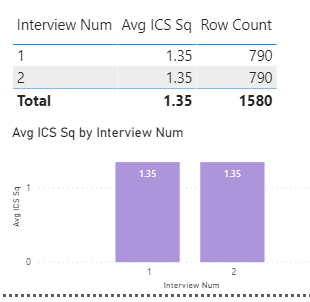
Results don’t get any more reliable than that. So, on to validity…
In terms of “accuracy”, we don’t know what each interviewee’s “true” score is, since there will be some natural variability in peoples’ scores. This variability could be due to additional consideration given/information acquired between the first and second interviews, peer and/or supervisor interactions, or factors completely unrelated to the relative merits of each policy (e.g., interviewee had an excellent breakfast and a traffic-free commute before the second interview, or conversely a loud argument with their surly teenage kid…). The key issue in assessing validity here is to make sure that the interviewer is not injecting their own variability/evaluation bias into the interview process. Fortunately, within the data we have an excellent control and experimental groups with which to explore this issue, since a little over 20% of the interviews were conducted by the same interviewer for both interview #1 and #2.
So, the first thing I looked at was whether average ICS Sq score differed by whether the interviews were conducted by the same interviewer or not:
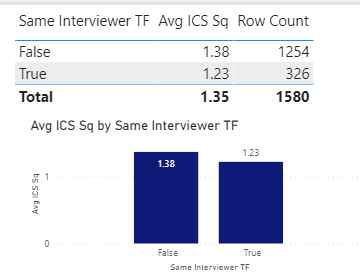
Average scores were slightly lower when the same person did both interviews, but the difference was not statistically significant.
So, what about if you break this down further by interview?
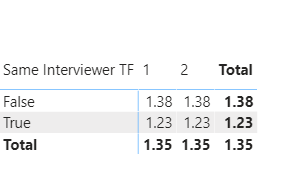
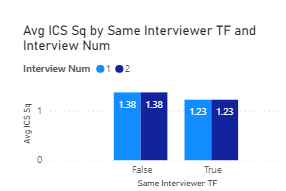
Exact same result as above, and not statistically significant.
Now, what about individual interviewer effects?:
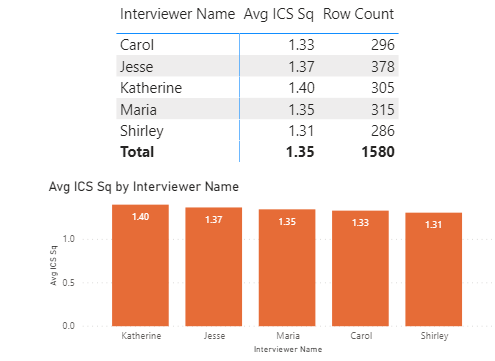
Some slight variability, but not even close to statistically significant.
And what if we break this down further by interview?
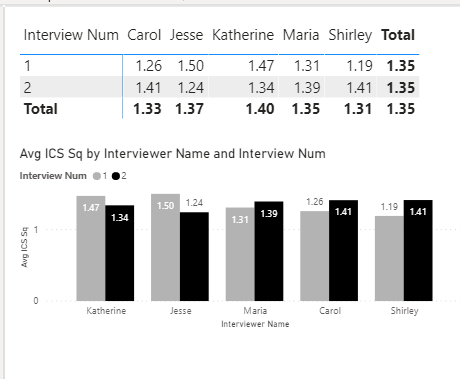
Again some variability observed across interviewers, but not approaching statistically significant.
So, we can sign off on validity as well, right? Not quite - we still have one more comparison to look at, and that’s the interaction between same/different interviewer and individual interviewers:
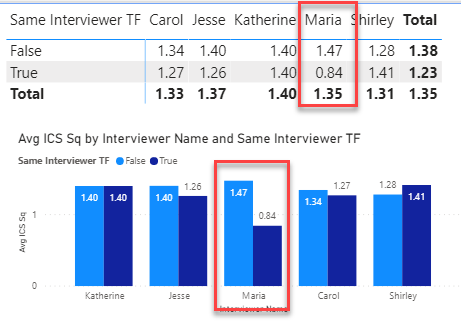
And this is where the red flags go up and the alarm bells go off, since Maria’s scores are exactly 75% higher when she is paired with another interviewer than when she is doing both interviews herself. This effect is statistically significant at the p = .06 level, and this is likely not a small sample size-driven finding, since Maria conducted 315 total interviews (2nd most) and for 25.5% of them she was the sole interviewer. When Maria is removed from the data and the analysis rerun, the differences do not approach statistically significant.
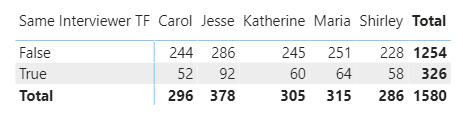
BOTTOM LINE
There is good reason to be concerned about the validity of Maria’s interviews. Before making any policy decisions based on this data overall, I would look at all the results both with and without Maria’s interviews included (i.e., include an interviewer slicer with which we can recalculate our report including and removing Maria).